Michael Ekstrand (Boise State University) 2022/11/20 13:40-14:40
The Complexity of Fairness in Information Access [スライド]
Ensuring that information access systems, such as recommender systems and search engines, are “fair” is a complex, multi-faceted problem. Significant progress has been made in recent years on identifying and measuring important forms of unfair recommendation and retrieval, but there are still many ways recommender systems can replicate, exacerbate, or mitigate potentially discriminatory harms that need careful study. These harms can affect different stakeholders — such as the producers and consumers of information, among others — in many different ways, including denying them access to the system’s benefits, misrepresenting them, or reinforcing unhelpful stereotypes.
In this talk, I will provide an overview of the landscape of fairness and anti-discrimination in information access systems, discussing both the state of the art in measuring relatively well-understood harms and new directions and open problems in defining and measuring fairness problems.
Bio
Michael Ekstrand is an associate professor of computer science at Boise State University, where he co-directs the People and Information Research Team. His research blends information retrieval, human-computer interaction, machine learning, and algorithmic fairness to try to make information access systems, such as recommender systems and search engines, good for everyone they affect. In 2018, he receieved the NSF CAREER award to study how recommender systems respond to biases in input data and experimental protocols and predict their future response under various technical and sociological conditions.
He received his Ph.D in 2014 from the University of Minnesota, building tools to support reproducible research and examining user-relevant differences in recommender algorithms with the GroupLens research group. He leads the LensKit open-source software project for enabling high-velocity reproducible research in recommender systems and co-created the Recommender Systems specialization on Coursera with Joseph A. Konstan from the University of Minnesota. He is currently working to develop and support communities studying fairness and accountability, both within information access through the FATREC and FACTS-IR workshops and the Fair Ranking track at TREC, and more broadly through the ACM FAccT community in various roles.
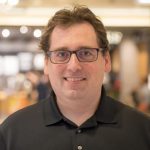
Denny Wu (University of Toronto / Vector Institute) 2022/11/21 13:00-14:00 13:30-14:30
Precise Analysis of Modern Machine Learning Models via Random Matrix Theory
The performance of many simple machine learning models can be precisely characterized in the proportional asymptotic limit (i.e., when the size of the dataset and the number of parameters jointly tend to infinity) using random matrix theoretical tools. Such precise analysis can reveal interesting phenomena that are also empirically observed in deep learning. In this talk I will introduce two examples. First we consider the generalized ridge regression estimator in the overparameterized regime, for which the asymptotic formulae of generalization error lead to surprising findings including: (i) the optimal ridge penalty can be negative, and (ii) regularization can suppress “multiple descent” in the risk curve. I will then discuss some practical implications such as the comparison of first- and second-order optimizers in neural network training. For the second part, we characterize the benefit of feature (representation) learning via gradient descent in a two-layer neural network. By studying the precise performance of kernel ridge regression on the trained features, we rigorously show that feature learning results in a considerable advantage over the initial random features model; this analysis also highlights the role of learning rate scaling in the initial phase of gradient descent training.
Bio
Denny Wu is a Ph.D. candidate at the University of Toronto and the Vector Institute for Artificial Intelligence, under the supervision of Jimmy Ba and Murat A. Erdogdu. Before that he obtained his bachelor’s degree from Carnegie Mellon University advised by Ruslan Salakhutdinov. He also maintains close collaboration with the University of Tokyo and RIKEN AIP, where he is currently working with Taiji Suzuki and Atsushi Nitanda.
Denny is interested in developing a theoretical understanding (e.g., optimization and generalization) of modern machine learning systems, especially overparameterized models such as neural networks, using tools from high-dimensional statistics.
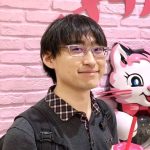
Luca Belli 2022/11/22 9:30-10:30
Responsible AI in Practice: Lessons from Experience at Scale
The responsible use of artificial intelligence, or RAI, is getting more attention and traction both in research and in the public discourse. What are the challenges of implementing research in practice? How does one translate ethics principle into product recommendations? What are the opportunities and limitations?
In this talk we are going to try answering those questions by looking at concrete examples, drawing from the past few years of practical experience with this work at scale in a worldwide company.
Bio
Luca is the co-founder and research lead of the Machine Learning Ethics Transparency and Accountability team at Twitter, that in joined in 2017. His research group is focused on studying the impact of Twitter’s machine learning systems, with special attention on feedback loops and algorithmic amplification.
Prior to that he was a Sr. Machine Learning engineer in adtech at Conversant, where he helped jump start their image recognition efforts. His first job was at Wolfram Alpha, where he worked in Boston for 3 winters as a Math Content Developer.
His background is in pure math, as he got a PhD in studying complex geometry in Rome Tor Vergata.
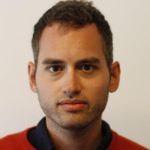